Five years ago it was impossible to imagine that Artificial Intelligence could handle business processes and tasks with the precision of a human specialist. Today, AI is capable of multiple business tasks, from automating data entry and generating client reports to personalizing marketing campaigns and forecasting sales trends. It can be embedded in CRMs, powering chatbots, enhancing cybersecurity, and even supporting decision-making by analyzing massive datasets in real time. Any business that wants to remain competitive in 2025 should embrace AI now.
How to Implement AI in Business Responsibly
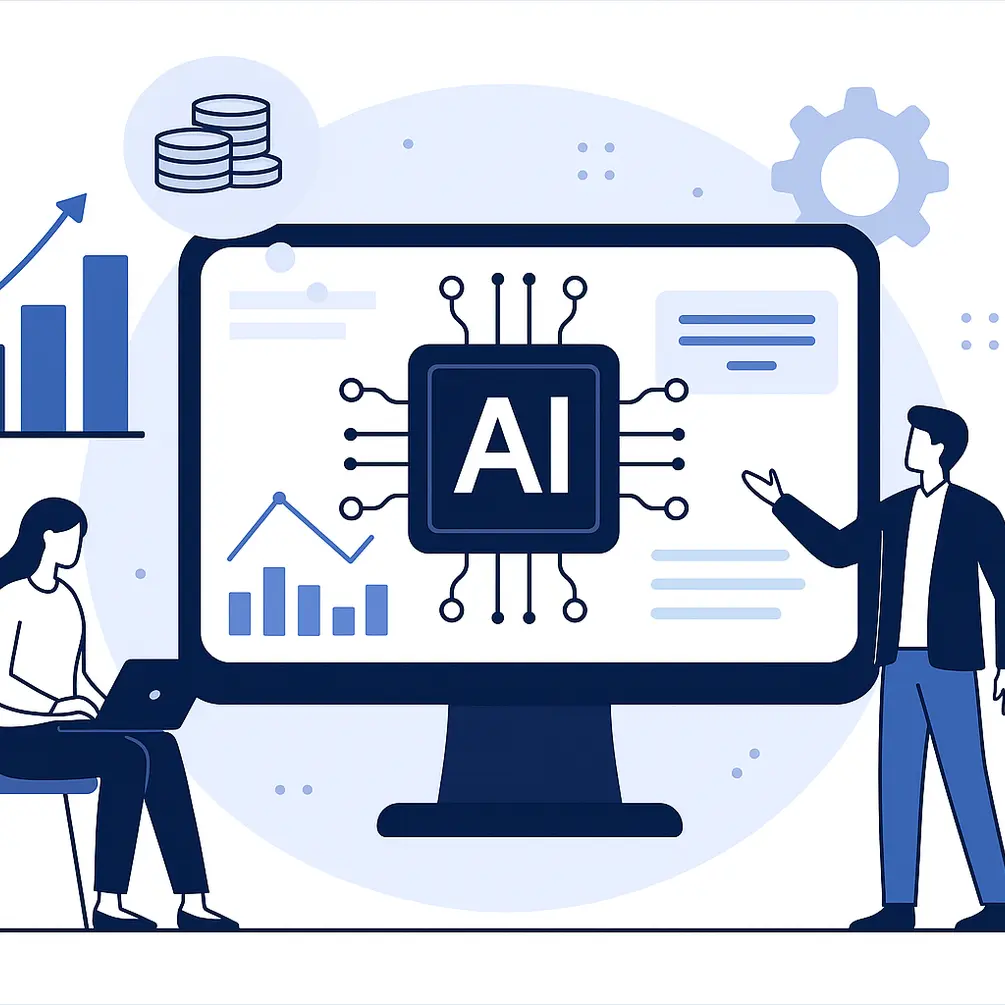
Artificial Intelligence is rapidly changing our approach to business and decision-making, opening up a world of great opportunities. What used to take hours now happens in seconds, and what once required entire teams can sometimes be done with the right AI tool and a well-written prompt. Global companies utilize AI to boost customer experience, optimize business processes, uncover hidden insights, and drive ROI.
Everyone is talking about the necessity to embed AI, but few think about the foundations that make it effective: clean data, data privacy, smart integration, and real human oversight. The essential question that remains is how to implement AI in business responsibly?
In this 2025 AI for Business Guide, the team at Drupfan how AI can solve business problems, as well as explains how to implement AI in your business responsibly.
What are the Benefits of Implementing AI in Business?
AI is not just reshaping workflows, it’s redefining how business gets done. Companies that treat it as another trend are already lagging behind. Those embedding it into core operations are cutting costs, moving faster, and pulling ahead. But let’s get specific. Below are the real, measurable benefits you'll get from implementing AI in business. Not the hype, but the hard wins that come from implementation done right.
Time Savings Where It Counts
AI never needs a break. While your team sleeps, models keep processing invoices, drafting proposals, triaging tickets, and analyzing customer behavior. That’s not a convenience, it’s hours back on the clock for every department.
The Adecco Group’s Global Workforce of the Future survey, based on responses from 35,000 workers across 27 major economies, found that AI saves employees an average of 1 hour per day, with 20% reporting savings of up to 2 hours daily thanks to the technology.
According to McKinsey's report on the Economic Potential of Generative AI, generative AI and other technologies could automate work activities that absorb 60 to 70 percent of employees' time today.
Faster Decision-Making
Forget gut instinct. When the stakes are high, AI models can analyze massive datasets in seconds, flagging trends, uncovering anomalies, and helping leaders make decisions with more confidence and less risk. Gartner projects that companies using AI for decision intelligence will execute 75% faster with fewer errors. That's the difference between being first to market and watching someone else take your share.
Personalized Customer Experiences
With behavioral data and predictive analytics provided after implementing AI in business, you can serve the right content, offer, or follow-up at exactly the right time. As a result, customers feel understood, not targeted or robotically chased. Salesforce found that 73% of customers expect companies to know who they are and what they need. AI is what makes that expectation scalable.
Stronger ROI from Marketing Spend
Stop wasting ad budget. AI tools can optimize creatives, track performance in real time, score leads, and retarget with uncanny precision. Instead of hunches, you're working with signals. Statista projects that AI-powered marketing will generate over $107 billion in global revenue by 2028. That’s because it works and smart marketers are already banking on it.
Reduced Human Error, Increased Compliance
AI is relentless about details. It catches what humans miss: miskeyed numbers, regulatory gaps, inconsistent records. It doesn’t get tired or overlook steps. In regulated industries, AI is becoming essential for audits, fraud detection, and reporting. Deloitte reports that AI-driven mapping can reduce compliance costs by 30% and improve accuracy by 25%.
Cost Reduction
Implementing AI in business means less waste, fewer manual tasks, tighter workflows. Accenture estimates that companies that successfully implement AI in business could increase profitability by an average of 38 percent by 2035.
Fun fact: Netflix saves over $1 billion a year using AI-powered recommendation systems. By analyzing viewer behavior, watch history, and engagement patterns, the platform serves up suggestions that keep people binge-watching and keep churn rates low. That’s not just good UX. That’s serious business intelligence in action.
→ Read more: AI in the Dev World: Hype, Help, or Hiring Hazard?
Work together with Drupfan to make AI practical, efficient, and useful for your business.
How to Choose the Right AI Model for Your Business?
You don’t start with the model. You start with the problem. Too many companies chase shiny algorithms without understanding what they actually need. Before you even think about machine learning frameworks or model training, take a step back. AI that works starts with strategy, not tech.
Step 1: Get Your Data House in Order
If your data is scattered, incomplete, or locked in legacy systems, AI won’t save you: it’ll struggle. Start by auditing the data you already have. Is it clean? Is it structured? Can it be accessed and processed in real time? AI feeds on quality inputs. Feed it junk, and it spits out junk faster.
Step 2: Clarify the Business Outcome
Do you want to reduce churn? Predict sales? Automate support? Enhance personalization? Be specific. A vague goal like “use AI to innovate” is a graveyard for resources. Instead, tie the model to a measurable business task, one that has clear KPIs and economic impact.
Step 3: Define the Task, Not the Tool
You’re not buying a hammer, you’re solving a very particular nail. Map your goal to the kind of task the AI needs to perform:
- Classification?
- Forecasting?
- Pattern recognition?
- Language generation?
- Recommendation?
This is your blueprint. From here, you choose the AI model to implement in your business.
Common AI Model Types and When to Implement Them in Your Business
Natural Language Processing (NLP)
If your business deals with massive volumes of unstructured text: emails, chat logs, customer reviews, NLP is your weapon. It parses, classifies, and extracts meaning from language. Use it for sentiment analysis, chatbot training, document classification, or summarization.
NLP model is ideal for:
- Automating customer service
- Mining insights from feedback
- Powering search functions
Just make sure your input data has linguistic diversity and represents real user queries, not sanitized mockups.
Deep Learning (DL)
Deep learning shines when your dataset is huge and your problem is complex. Think image recognition, fraud detection, or predictive maintenance with IoT inputs. DL models (like CNNs or LSTMs) can process thousands of variables, spot non-obvious patterns, and get smarter over time—if you train them properly.
Use DL if you need:
- High accuracy over time
- Real-time inference at scale
- Analysis of audio, video, or visual inputs
But watch out: it’s compute-hungry and expensive to train. Don’t reach for deep learning unless your business genuinely needs it.
Machine Learning (ML)
Don’t overlook the classics. For problems like churn prediction, lead scoring, inventory forecasting, or risk modeling, a well-tuned logistic regression or random forest often outperforms overhyped neural nets. They’re explainable, cheaper to run, and faster to deploy.
Use ML when:
- You want interpretable results
- You have smaller datasets
- Speed and cost matter more than ultra-high accuracy
Traditional ML is the right call for 80% of use cases—especially if you’re new to AI.
Generative AI
Generative AI is already reshaping customer support, marketing ops, product design, and internal documentation. Trained on massive datasets, these models can generate text, images, audio, code, or even synthetic data that mimic real-world patterns with shocking accuracy.
Use Generative AI when:
- You need high-quality content at scale (emails, summaries, reports)
- Your teams are bogged down by repetitive creative or writing tasks
- You want to prototype fast—copy, visuals, interfaces, mock data
Generative AI works best when paired with human oversight.
So, How Do You Choose Which AI Model to Implement in Business?
The answer is: match the AI model type to the task. Strike a balance between intelligence and practicality. And above all, stay brutally honest about your internal capabilities. The right model isn’t always the trendiest. It’s the one your team can train, deploy, monitor, and actually extract value from without stalling three months into development. If a simpler supervised learning model solves the problem, use it. If your use case needs fine-tuned transformers, invest the time and talent to get them right. Either way, your goal isn’t to impress your CTO or mirror what a Big Tech case study did last year. Your goal is to solve a business problem and evolve your business.
If you’re serious about implementing AI in business, involve AI experts that can ensure the chosen AI tool is aligned with your data strategy and business objectives. Such specialists can provide expert guidance not only on model selection but also on its integration into your business processes. Think of it like bringing in a financial advisor before investing big money. Hiring AI experts early can save you months of rework, budget drain, and the kind of mistakes that don’t show up until it’s too late.
→ Read more: AI vs. Real Developers: The Battle of Brains and Bots
How to Implement AI in Business Processes?
Artificial Intelligence is a capability that must be planned, integrated, trained, and continuously refined. The companies that have already successfully embraced AI methodically embedded it into processes that matter, using data they trust, and aligning it with outcomes that move the business forward. Here's a step-by-step roadmap to help you implement AI in your business:
Step 1: Start with a Business-First Mindset
AI is not the strategy. It’s a tool to execute strategy better, faster, and at scale. Begin by identifying business processes where AI can create measurable impact, whether it’s reducing costs, increasing speed, improving accuracy, or enhancing the customer experience. Think predictive maintenance, lead scoring, invoice automation, fraud detection, or dynamic pricing.
Avoid the trap of "AI for AI’s sake." You’re not here to impress stakeholders with trends. You’re implementing AI in business to increase revenue, protect margins, or outpace competitors.
Step 2: Conduct a Data Audit
AI is only as good as the data feeding it. Before you build or buy any models, evaluate the data you have across your systems.
Ask:
- Is the data clean, complete, and accurate?
- Do you have the historical depth needed for training models?
- Can the data be accessed and integrated securely across teams?
Most AI initiatives fail because the data layer is weak. This is the time to invest in data engineering and governance. Without reliable data pipelines, your models will underperform or flat-out misfire.
Step 3: Identify the Right Use Cases
Not every process is ready for AI, and not every model will yield ROI. Narrow your focus to high-impact, high-frequency processes that:
- Involve repetitive decisions
- Depend on pattern recognition or prediction
- Require real-time responsiveness
Prioritize use cases that are easy to pilot but valuable enough to justify scaling. Example: AI-based email triage for customer service, or churn prediction in subscription models. These are mature, proven applications with fast time-to-value.
Step 4: Choose the Right AI Approach
Now that you know the task, pick the tool. This is where AI model selection comes in. You might need:
- Machine Learning (ML): For forecasting, classification, scoring, etc.
- Natural Language Processing (NLP): For analyzing text, automating emails, building chatbots.
- Deep Learning (DL): For complex tasks and detecting patterns in massive, unstructured datasets.
- Generative AI: For creating text, marketing copy, code, or design variations.
You can build custom models with platforms like TensorFlow or PyTorch, or integrate pre-trained APIs from providers like OpenAI, Google Cloud, or AWS. The decision depends on your use case, timeline, budget, and in-house capabilities.
Step 5: Assemble the Right Team
Successful AI implementation isn’t an IT-only initiative. You’ll need:
- Data Engineers to prepare and pipeline data
- ML Engineers/Scientists to build and tune models
- Business Analysts to tie AI outcomes to KPIs
- Project Managers to keep it all moving
If you lack internal expertise, consider working with a specialized AI consultancy. What matters is that your team can move from prototype to production without losing momentum.
Step 6: Start with a Pilot, Then Scale
Don’t attempt an enterprise-wide rollout from day one. Build a contained pilot with clear success metrics. Monitor model performance, business impact, and user adoption. Collect feedback from real users and be ready to tweak the process or retrain the model.
Once the pilot proves itself, scale iteratively. Build integrations, automate retraining cycles, and develop dashboards for explainability and performance monitoring.
Step 7: Focus on Governance and Ethics
AI doesn’t eliminate risk, it shifts it. You’ll need strong governance around:
- Data privacy and consent
- Bias and fairness in decision-making
- Model explainability and auditability
- Regulatory compliance
Assign ownership, document model logic, and set thresholds for intervention. CEOs should treat AI ethics like cybersecurity: not optional, and definitely not someone else’s problem.
Step 8: Build a Feedback Loop
AI isn’t static. It requires ongoing learning. Embed feedback from users, monitor model drift, and invest in MLOps tools to manage versioning, retraining, and deployment. The most successful AI-driven companies treat their models like products with updates, metrics, and accountability.
Challenges You Can Face While Implementing AI in Business
Implementing AI in business is a strategic shift, and like any shift, it comes with friction. Misjudging these challenges can burn through budgets, derail timelines, and leave leadership questioning the entire initiative. Here are the most critical obstacles you’ll face when integrating AI into your business and how to address them head-on.
1. Poor Data Quality and Fragmentation
AI runs on data. If your data is scattered across systems, full of duplicates, or missing key fields, your model performance will suffer, or worse, produce misleading results. Fragmented data silos also prevent cross-functional insights that AI could surface.
The fix: Start with a full data audit before any model work begins. Invest in data engineering, clean up legacy systems, and unify your datasets into a centralized, structured, and accessible environment. Set up pipelines to feed AI models fresh, relevant data continuously. No model will outrun a bad foundation.
2. Lack of Clear Business Goals
“We want to use AI” is not a strategy. Many companies jump into implementation without clearly defining the problem they’re trying to solve. That leads to misaligned efforts, bloated prototypes, and zero ROI.
The fix: Tie AI to specific business outcomes. Whether it's reducing churn, increasing support resolution speed, or forecasting supply chain risk: set a measurable target. Work backward from the goal to select use cases, data inputs, and models. If it doesn’t drive revenue, reduce cost, or improve experience, it’s a distraction.
3. Skills and Talent Gaps
Building, training, and maintaining AI models isn’t the same as installing a CRM. You need specialists: data scientists, ML engineers, MLOps experts, and many companies underestimate the complexity of hiring and integrating them into traditional IT or product teams.
The fix: Start by upskilling internal teams on AI fundamentals, then bring in external consultants or hire experienced AI engineers for critical phases. If you’re new to AI, pilot with a vendor or partner who can bring tools, talent, and experience without locking you into long-term dependency.
4. Integration with Legacy Systems
Your AI solution might be ready, but if your core platforms—ERPs, CRMs, or databases—can’t interface with it cleanly, you’ll hit roadblocks. Legacy systems weren’t built to speak the same language as real-time AI pipelines.
The fix: Use APIs, middleware, or data virtualization to bridge the gap. Consider modular AI tools that sit alongside existing infrastructure rather than rebuilding everything. Your goal is a gradual, non-disruptive evolution—not a rip-and-replace project that stalls operations.
5. Model Transparency and Trust
When AI outputs affect customers, employees, or financial decisions, the “black box” problem becomes real. If your team doesn’t understand how a decision was made, they’re less likely to trust or act on it.
The fix: Favor explainable AI models, especially in regulated industries. Document model logic, assumptions, and edge cases. Build dashboards that show confidence scores and decision paths. Train business stakeholders on how AI fits into workflows so they become owners, not skeptics.
AI can unlock real competitive advantages but only for businesses that respect the complexity behind the hype. Handle the challenges early, and you set yourself up for scalable, reliable success. Ignore them, and you risk becoming another “AI failed us” cautionary tale.
Implementing AI in Business Responsibly: Final Thoughts
AI isn’t a silver bullet, it’s a system that reflects the data, values, and intent behind it. Responsible implementation means more than compliance checkboxes. It’s about building systems that are transparent, fair, and accountable. It means questioning where automation makes sense, where human oversight is essential, and how decisions affect real people. Businesses that get this right don’t just move faster, they build trust. They scale without cutting ethical corners. As AI becomes more powerful, the edge won’t belong to those who rush, but to those who implement with purpose, clarity, and long-term vision.